Najnowsze trendy i zmiany paradygmatów w branży patologii cyfrowej
Piśmiennictwo
- Kannan S. et al.: Segmentation of glomeruli within trichrome images using deep learning. „Kidney International Reports”, 2019, 4, 7, 955-962.
- Martin D.R. et al.: A Deep Learning Convolutional Neural Network Can Recognize Common Patterns of Injury in Gastric Pathology. „Archives of Pathology & Laboratory Medicine”, 2019.
- Guo X. et al.: Liver Steatosis Segmentation With Deep Learning Methods. 2019 IEEE 16th International Symposium on Biomedical Imaging (ISBI 2019). IEEE, 2019.
- Yu Y. et al.: Deep learning enables automated scoring of liver fibrosis stages. „Scientific Reports”, 2018, 8, 1, 1-10.
- Kapil A. et al.: Deep semi supervised generative learning for automated tumor proportion scoring on NSCLC tissue needle biopsies. „Scientific Reports” 2018, 8, 1, 1-10.
- Aprupe L. et al. Robust and accurate quantification of biomarkers of immune cells in lung cancer micro-environment using deep convolutional neural networks. „PeerJ”, 2019, 7, e6335.
- Heinemann F. et al.: Deep neural network based histological scoring of lung fibrosis and inflammation in the mouse model system. „PloS one”, 2018, 13, 8.
- Xie Y. et al.: Deep Learning for Muscle Pathology Image Analysis. Deep Learning and Convolutional Neural Networks for Medical Imaging and Clinical Informatics. Springer, Cham, 2019, 23-41.
- Sing T. et al.: A deep learning-based model of normal histology. „bioRxiv”, 2019, 838417.
dr n. wet. Aleksandra Żuraw
Charles River Laboratories
22022 Trans-Canada Hwy,
Senneville, QC H9X 1C1, Kanada
Mogą zainteresować Cię również
Znajdź swoją kategorię
2814 praktycznych artykułów - 324 ekspertów - 22 kategorii tematycznych
Weterynaria w Terenie

Praktyka w terenie – jak zabezpieczyć się na wypadek stanów zagrożenia życia lub zdrowia zwierzęcia
Piśmiennictwo mec. Anna SłowińskaVox Poland Pomoc PrawnaSzczecin Facebook0Tweet0LinkedIn0

Choroby racic jako ważna przyczyna brakowania krów w stadzie bydła mlecznego
Piśmiennictwo dr n. wet. Małgorzata Dziekiewicz-Mrugasiewieczlek. wet. Maciej PerzynaKatedra Chorób Dużych Zwierząti Klinika Instytutu Medycyny WeterynaryjnejSGGW w Warszawie Facebook0Tweet0LinkedIn0
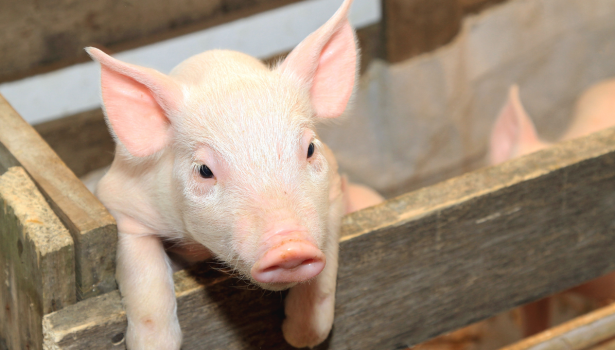
Czynniki wpływające na kolonizację mikrobiomu i dojrzałość układu pokarmowego prosiąt oraz jego prawidłowe funkcjonowanie
Piśmiennictwo dr inż. Piotr Nowak Facebook0Tweet0LinkedIn0

Opieka stomatologiczna nad starszymi końmi
Piśmiennictwo lek. wet. Katarzyna FerenzGabinet weterynaryjny Końskie Zdrowie we Wrocławiu Facebook0Tweet0LinkedIn0

Praktyka w terenie – jak zabezpieczyć się na wypadek stanów zagrożenia życia lub zdrowia zwierzęcia
Piśmiennictwo mec. Anna SłowińskaVox Poland Pomoc PrawnaSzczecin Facebook0Tweet0LinkedIn0

Wiek ma ogromne znaczenie podczas stawiania diagnozy
Lek. wet. Justyna Domagała tytuł inżyniera zootechniki uzyskała w 2016 r., a tytuł lekarza weterynarii – w 2019 r. na Uniwersytecie Przyrodniczym we Wrocławiu. Po studiach swoją wiedzę poszerzała podczas stażu w Szpitalu dla Koni Equivet, w którym później uzyskała zatrudnienie. W latach 2021-2024 swoją pracę skupiała na internistycznym leczeniu koni oraz pogłębianiu wiedzy na […]

Echa 32. Kongresu Bujatrycznego w Cancun
Profilaktyka Kongres był również okazją do zaprezentowania kilku przełomowych produktów immunologicznych, które mają szansę wprowadzić na nowe tory prewencję znanych od lat jednostek chorobowych, sprawiających wiele kłopotów z punktu widzenia odchowu cieląt czy rozrodu. Mowa tu konkretnie o trzech nowych szczepionkach mających zastosowanie w profilaktyce Mycoplasma bovis, Cryptosporidium parvum czy wirusowej biegunki bydła (BVD). Nie […]